Inventory Forecasting Guide: Methods, Example, and Formulas
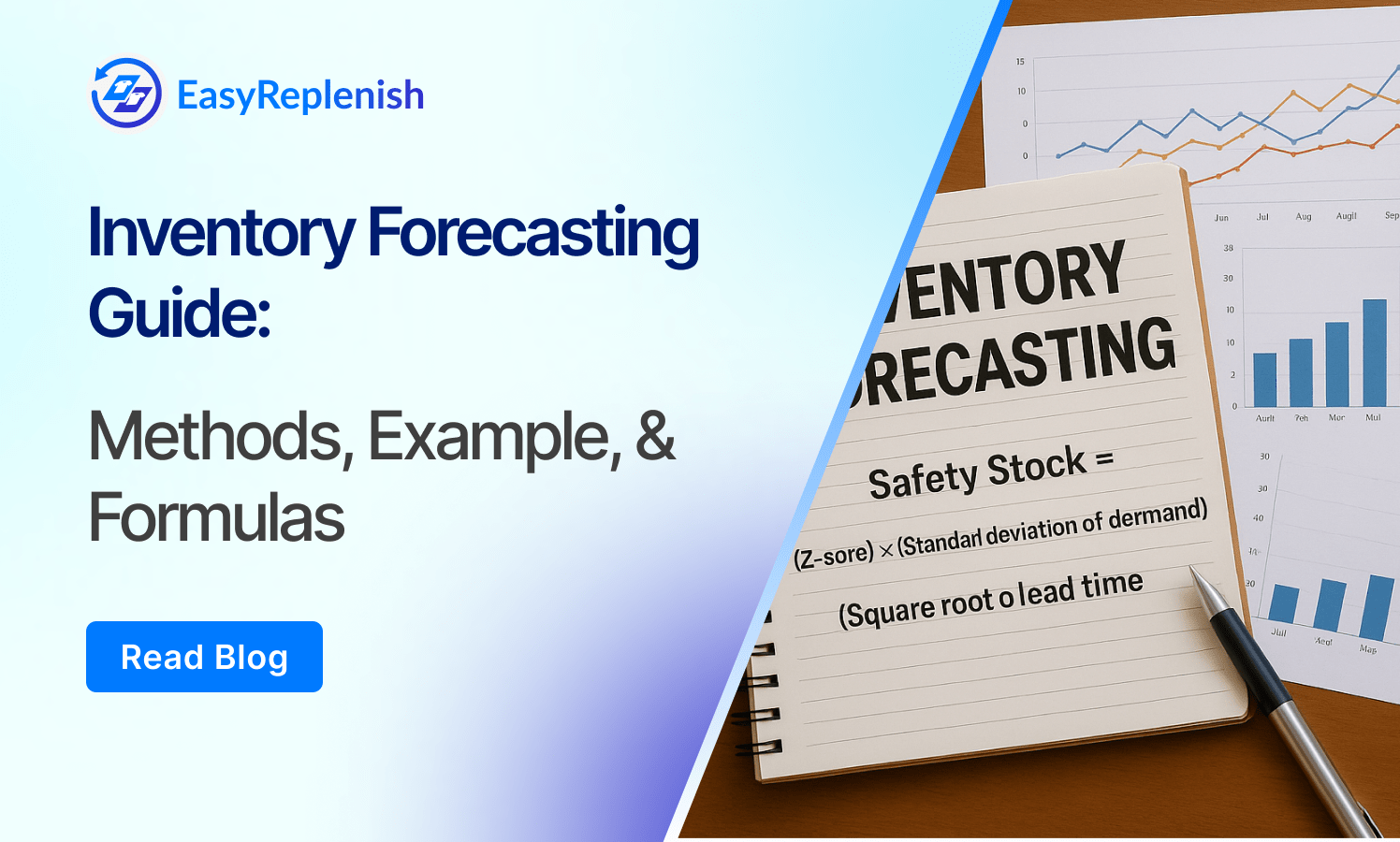
Introduction
Inventory forecasting isn’t just a supply chain function — it’s a growth lever. Whether you're running a DTC eCommerce brand, managing B2B distribution, or operating a multi-warehouse retail chain, accurate inventory forecasts mean fewer stockouts, optimized working capital, and better customer experience.
In a world where demand shifts overnight and supply chains are anything but predictable, guessing how much stock you need is expensive. Overstock leads to cash being tied up in slow-moving inventory. Understock leads to missed sales and frustrated customers. Forecasting fixes that — if done right.
This guide breaks down inventory forecasting into practical, tactical insights so you can apply it to your business immediately — no jargon, no fluff.
What is Inventory Forecasting?
Inventory forecasting is the process of predicting how much stock you’ll need to meet future customer demand. It uses historical sales data, market trends, lead times, and other variables to ensure you have the right products, in the right quantities, at the right time.
But it’s more than just estimating demand — it’s a strategic function that connects operations, procurement, finance, and even marketing. A solid forecast informs how much inventory to order, when to order it, how much safety stock to keep, and how to plan around promotions or seasonal peaks.
Modern inventory forecasting combines both data-driven models and business context. It’s not about being 100% accurate — it’s about being accurate enough to reduce risk and make smarter decisions across the supply chain.
Inventory Forecasting vs Replenishment: What’s the Difference?
Inventory forecasting and replenishment are closely linked, but they serve distinct roles in inventory management. Understanding this difference helps avoid stockouts, overstocking, and wasted capital.
1. Forecasting = Planning Ahead
Forecasting is the proactive process of predicting future demand based on historical sales, seasonality, market trends, and lead times. It helps answer:
“How much stock will I need — and when?”
2. Replenishment = Reacting to Demand
Replenishment is the reactive process of restocking inventory when it drops below a defined threshold. It focuses on:
“When should I reorder — and how much?”
Example in Action
If you're selling skincare online, forecasting tells you Q4 demand for your best-selling serum will jump 40%. Replenishment ensures new stock is ordered when levels drop below 200 units — before you run out.
Why It Matters
Forecasting without solid replenishment creates outdated stock plans. Replenishment without accurate forecasts leads to reactive, costly decisions. When both are aligned, you build a lean, responsive inventory system that reduces costs and improves customer satisfaction.
Types of Inventory Forecasting: Choosing the Right Model for Your Business
There’s no one-size-fits-all approach to forecasting. Different products, sales cycles, and industries require different forecasting methods. Choosing the right type of inventory forecasting is essential for accuracy and profitability.
According to a McKinsey report, companies that apply advanced forecasting models can reduce inventory costs by 10–30% while improving service levels by up to 20%.
Here are the four key types of inventory forecasting used in modern supply chains:
1. Quantitative Forecasting (Data-Driven, Proven, Scalable)
Quantitative forecasting uses historical sales data and statistical algorithms to predict future demand. It’s the backbone of modern inventory planning systems — and ideal for businesses with consistent sales patterns across multiple SKUs.
Best for:
- Products with steady demand or strong seasonality
- Businesses with at least 12–24 months of clean sales data
- Use cases where scalability and repeatability matter
Common models include:
- Moving Average: Smooths short-term fluctuations to find a demand baseline
- Exponential Smoothing: Weighs recent data more heavily for agile adjustments
- Linear Regression: Useful when demand correlates with variables like pricing or advertising spend
Bonus: Quantitative models integrate well with forecasting software and ERP systems, enabling automation and faster decision-making.
2. Qualitative Forecasting (Insight-Driven, Flexible, Essential for New Products)
When you lack reliable historical data — or you're entering a new market — qualitative forecasting fills the gap. It relies on expert opinion, customer feedback, and market research to build directional forecasts.
Best for:
- New product launches without sales history
- Market disruptions (e.g. pandemic-era demand shifts)
- Demand spikes driven by unpredictable external events
Common sources of input:
- Sales team forecasts
- Supplier and distributor insights
- Customer surveys, social listening, and competitor analysis
- Executive judgment and market intelligence reports
Pro tip: Use qualitative forecasting alongside early performance data to adjust faster. It’s not about precision — it’s about direction.
3. Short-Term vs. Long-Term Forecasting (Tactical vs Strategic Planning)
Both timeframes matter — but they serve different purposes.
Short-term forecasting (days to weeks) helps with:
- Replenishment planning
- Staffing and shift scheduling
- Handling flash sales, promotions, or upcoming holidays
Long-term forecasting (months to a year) supports:
- Strategic procurement and budgeting
- Production planning with long lead times
- Seasonal product decisions and contract negotiations
Why it matters:
Short-term forecasts reduce day-to-day risk. Long-term forecasts drive big-picture profitability. The most resilient supply chains run both simultaneously — often with different models for each.
4. Static vs. Dynamic Forecasting Models (How Adaptable is Your Forecast?)
Forecasting models can either stay fixed or adapt continuously — and your choice affects how you respond to demand shifts.
- Static forecasting uses fixed assumptions (e.g., average monthly sales, static lead time). It’s easier to implement and maintain but struggles with fast-changing environments.
- Dynamic forecasting pulls in real-time data like website traffic, promotional calendars, supply chain disruptions, and external trends (e.g., weather or economic indicators). These models adjust constantly, making them ideal for industries like fashion, electronics, or FMCG.
Example:
A fashion brand using real-time influencer campaign data to adjust demand for specific SKUs within hours of posting.
When to use:
- Static models: when demand is predictable and stable
- Dynamic models: when agility is critical and data flows are live
Tip: Even if you start with static models, aim to transition to dynamic systems as your data infrastructure matures.
5. Blending Models = Better Forecasts
The most effective inventory strategies don’t rely on one type alone. Mature businesses use hybrid models — layering quantitative forecasts with qualitative insights, and balancing short-term agility with long-term planning.
The goal isn’t perfect accuracy. The goal is consistent, informed action that reduces stockouts, trims waste, and supports profitable growth.
Benefits of Inventory Forecasting: Strategic Wins Beyond Stock Planning
Here are the key benefits of inventory forecasting — beyond the usual "avoiding stockouts" narrative:
1. Lower Inventory Carrying Costs
Every unsold unit ties up working capital, requires storage, insurance, and risks obsolescence. With better forecasting, you hold only what you need — nothing more.
Impact: Companies can reduce carrying costs by up to 25% by aligning inventory levels with demand patterns.
2. Fewer Stockouts and Lost Sales
Forecasting minimizes the risk of running out of high-demand SKUs. It allows teams to prepare ahead for seasonal peaks, promotions, or supply delays.
Impact: A 1% reduction in stockouts can improve revenue by 2–5%, especially in eCommerce and retail.
3. Higher Inventory Turnover
Forecasting keeps inventory moving — reducing dwell time on shelves and improving turnover ratios. High turnover equals better cash flow and fewer write-offs.
Impact: Businesses with optimized forecasting often see inventory turnover increase by 15–30% within 6–12 months.
4. Improved Supplier Relationships
Sharing accurate forecasts with suppliers helps them plan production and fulfillment, reducing lead time variability and last-minute costs. Some suppliers even offer better pricing or terms when they can plan ahead with your forecasts.
Impact: Stable, forecast-backed procurement improves vendor trust and unlocks bulk or early-order discounts.
5. More Confident Financial Planning
Forecasting connects inventory to financial operations. When you can predict demand, you can budget more accurately, optimize cash flow, and prevent excess capital from sitting in warehouses.
Impact: CFOs use forecast data to model inventory ROI, working capital cycles, and EBITDA impact — not just stock levels.
6. Agile Demand-Driven Operations
Modern supply chains are volatile. Forecasting enables more flexible, data-informed responses to market shifts — whether it’s adjusting production, rerouting inventory, or preparing for disruptions.
Impact: Agile, forecast-led businesses bounce back 40–60% faster from demand shocks or supply disruptions.
7. Smarter Merchandising and Promotions
Good forecasting helps teams time promotions, bundle products, and run campaigns based on projected inventory flow — not guesswork.
Example: If you know your top SKUs will slow down in Q1, you can pre-plan bundles or flash sales to move inventory strategically.
8. Better Warehouse Optimization
Accurate forecasting reduces dead stock and improves warehouse slotting, space utilization, and labor efficiency. You spend less time managing excess — and more time fulfilling orders fast.
9. Stronger Omnichannel Fulfillment
For brands selling across retail, eCommerce, and marketplaces, forecasting enables better stock allocation across all channels — so you don’t run out on one and overstock another.
10. Scalable Growth with Less Risk
Ultimately, forecasting gives you the confidence to scale. Whether launching new products, entering new markets, or onboarding new fulfillment partners, accurate forecasts de-risk your growth roadmap.
How to Choose the Right Inventory Forecasting Method
Choosing the right inventory forecasting method isn’t about picking the most advanced model — it’s about choosing the one that fits your business model, data maturity, and operational needs.
Here’s a practical framework to help you make the right decision:
1. Assess Your Data Quality
Your forecasting method is only as good as your data. Ask:
- Do you have at least 12 months of historical sales data?
- Is your data clean, consistent, and tied to specific SKUs or locations? If yes, quantitative models like moving averages or regression will work. If not, start with a blended approach that includes qualitative forecasting.
2. Understand Your Demand Patterns
- Stable demand: Go with simple time-series models (e.g., moving average).
- Seasonal demand: Use models that factor seasonality (e.g., exponential smoothing with seasonal components).
- Volatile demand or new products: Layer in qualitative data and short-term dynamic models.
3. Map Forecasting to Business Goals
- If your goal is cost reduction, focus on optimizing inventory turnover with quantitative forecasting.
- If you’re launching new SKUs or entering new markets, lean into qualitative inputs and agile, short-term models.
4. Consider Operational Constraints
Your forecasting method should work within your supply chain realities:
- Long lead times? Use long-term forecasting.
- Highly perishable products? Prioritize short-term precision.
- Limited planning resources? Start with low-maintenance, semi-automated models.
5. Don’t Overcomplicate It
Advanced doesn’t always mean better. Machine learning models can sound impressive, but without the right infrastructure and skill sets, they often produce unreliable or hard-to-interpret results.
Simpler models — like moving averages, exponential smoothing, or regression — often outperform AI-based systems when data is limited or noisy. The key is to choose a method your team can understand, validate, and refine consistently. Complexity without clarity is a liability in forecasting.
6. Test, Measure, Improve
Inventory forecasting isn’t a set-it-and-forget-it task — it’s a continuous loop. Monitor forecast accuracy using metrics like MAPE (Mean Absolute Percentage Error), forecast bias, and service levels.
But don’t stop there. Analyze where the model over- or under-performs, then adjust assumptions, recalibrate model parameters, or add new data inputs. Treat forecasting as a living system that evolves as your business and market dynamics change.
10 Inventory Forecasting Tips and Strategies for Better Accuracy
Even with the right model in place, execution is everything. Here are 10 practical forecasting strategies used by high-performing inventory teams:
1. Segment Your Inventory by SKU Behavior
Don’t treat all SKUs the same. Use ABC or XYZ analysis to categorize SKUs by sales volume or variability — and apply forecasting methods accordingly.
2. Use Rolling Forecasts Instead of Static Plans
Demand changes fast. A rolling forecast (e.g., updated monthly for the next 3–6 months) is far more reliable than a fixed 12-month plan.
3. Incorporate Real-Time Signals
Leverage website traffic, sales velocity, backorders, and channel-specific demand signals to adjust forecasts on the fly — especially during campaigns or peak seasons.
4. Align Forecasting with Marketing and Sales
Marketing runs a promotion? Sales teams hear buyer hesitation? Feed that intel into your forecast adjustments before it’s reflected in data.
5. Track Forecast Accuracy (and Learn from Misses)
Use metrics like MAPE (Mean Absolute Percentage Error), forecast bias, or service level hit rates to measure performance — then run root cause analysis on misses.
6. Automate Low-Value Forecasting
Use your inventory management system or ERP to auto-forecast stable, high-volume SKUs — freeing time for your team to focus on high-impact exceptions.
7. Plan for Lead Time Variability
Build lead time buffers into your model, especially if you rely on overseas suppliers or custom manufacturing.
8. Apply Safety Stock — Intelligently
Don’t overbuffer everything. Calculate safety stock based on demand variability, service level targets, and lead time volatility — not gut feeling.
9. Use Forecast Scenarios
Build best-case, worst-case, and base-case scenarios to stay agile. Scenario planning helps you make smarter decisions when uncertainty hits.
10. Treat Forecasting as a Cross-Functional Discipline
Involve supply chain, finance, marketing, and product teams in the process. Forecasting accuracy improves dramatically when all stakeholders contribute context and constraints.
Inventory Forecasting Formulas
Effective inventory forecasting relies on a few essential formulas that help predict future demand, optimize stock levels, and improve overall supply chain efficiency. Below are some key formulas that can be used in inventory forecasting:
1. Moving Average Formula
The moving average formula helps smooth out sales fluctuations by averaging demand over a set number of periods. It’s useful when demand is relatively stable, with no seasonal spikes.
Formula:
Moving Average = (Total demand over a set number of periods) ÷ (Number of periods)
Example:
If you want to calculate a 3-month moving average of product sales:
- January sales = 1,000 units
- February sales = 1,200 units
- March sales = 1,100 units
The moving average would be:
(1,000 + 1,200 + 1,100) ÷ 3 = 1,100 units
This means that the predicted demand for the next period is 1,100 units.
2. Exponential Smoothing Formula
Exponential smoothing is used to forecast demand while giving more weight to recent sales data, making it useful when you want to account for recent trends.
Formula:
Next Forecast = (Smoothing Constant) × (Actual Demand) + (1 - Smoothing Constant) × (Previous Forecast)
Example:
If the smoothing constant (α) is 0.3, the last forecast was 1,100 units, and actual demand was 1,200 units, the new forecast would be:
0.3 × 1,200 + 0.7 × 1,100 = 1,130 units
This means the predicted demand for the next period is 1,130 units.
3. Seasonal Index Formula
The seasonal index formula helps adjust your forecast based on regular, recurring demand patterns such as holidays or weather-related changes.
Formula:
Seasonal Index = (Demand during peak season) ÷ (Average demand over time)
Example:
If the average sales are 1,000 units and sales during the peak season are 1,500 units, the seasonal index would be:
1,500 ÷ 1,000 = 1.5
This means demand during the peak season is 1.5 times the average demand.
4. Reorder Point Formula
The reorder point tells you when to place a new order for stock based on the time it takes for new inventory to arrive and the rate of product sales.
Formula:
Reorder Point = (Average demand per period) × (Lead time in periods)
Example:
If the average demand is 200 units per week, and it takes 3 weeks for new stock to arrive, the reorder point would be:
200 × 3 = 600 units
This means you need to reorder when you have 600 units left to avoid stockouts.
5. Safety Stock Formula
Safety stock is the extra inventory you keep on hand to protect against unexpected demand spikes or supply chain disruptions.
Formula:
Safety Stock = (Z-score) × (Standard deviation of demand) × (Square root of lead time)
(Here, Z-score represents the service level you want — for example, a Z-score of 1.96 corresponds to a 95% service level.)
Example:
If the standard deviation of demand is 50 units, the lead time is 4 weeks, and you want to maintain a 95% service level, the safety stock would be:
1.96 × 50 × √4 = 196 units
This means you should keep 196 units as safety stock to avoid running out.
6. Economic Order Quantity (EOQ)
The Economic Order Quantity (EOQ) formula helps determine the most cost-effective order size by balancing ordering and holding costs.
Formula:
EOQ = √(2 × Demand × Ordering cost ÷ Holding cost)
Example:
If you order 10,000 units annually, the ordering cost is $50 per order, and the holding cost is $2 per unit per year, the EOQ would be:
√(2 × 10,000 × 50 ÷ 2) = 707 units
This means you should order 707 units at a time to minimize costs.
Inventory Forecasting Example
To bring all the theory into action, let’s walk through a real-world example of how inventory forecasting works for a company selling fashion apparel.
Scenario: Fashion Retailer – Spring/Summer 2025 Forecasting
Retail Channels:
- 5 physical stores
- Online website
- Marketplaces (Amazon + Myntra)
Forecast Objective:
Estimate April–June 2025 demand for top 3 SKUs of the Spring/Summer collection, factoring in:
- Historical seasonality
- Growth trends
- Channel-level performance
- Planned influencer campaigns
Step 1: Historical Monthly Sales (Units Sold)
Step 2: Growth & Seasonality Insights
- Annual growth trend (based on YoY changes):
SKU A: ~15% • SKU B: ~12% • SKU C: ~20% - April–May consistently sees a 20–25% spike due to weather + early summer promotions.
- Influencer campaign planned for May 2025, expected to drive additional +15% uplift.
Step 3: Forecast Using Holt-Winters (Smoothed and Adjusted)
Smoothed Baseline Projection (Pre-Campaign):
Adjusted Forecast with Marketing Impact (May only):
Step 4: Channel-Level Distribution
Based on historical sell-through % across channels:
Step 5: Post-Season Evaluation Plan (July 2025)
- Compare actual vs forecast using:
- MAPE (Mean Absolute Percentage Error)
- Forecast Bias (Over/Under Forecasting)
- MAPE (Mean Absolute Percentage Error)
Example:
Insights:
- SKU A slightly over-forecasted in May due to overestimating influencer ROI
- SKU C under-forecasted — actual sales surged post social mentions in June
- Adjust campaign sensitivity and trend weights for next year
Conclusion
Inventory forecasting is a powerful tool that drives operational excellence, improves cash flow, and enhances customer satisfaction. Whether you're an eCommerce business, a brick-and-mortar retailer, or a B2B company, choosing the right forecasting method — and continuously refining it — is key to maintaining an agile, data-driven supply chain.
By applying the right methods, gathering continuous feedback, and integrating cross-functional insights, your inventory forecasting will not only meet today’s demand but also set you up for long-term success.
Frequently Asked Questions (FAQs)
Q1. What is the best inventory forecasting method for my business?
The best inventory forecasting method depends on your business type, sales patterns, and industry. If your demand is relatively stable, Moving Average might be ideal. For businesses with fluctuating demand or clear seasonal trends, Exponential Smoothing or Seasonal Index may be more suitable. For businesses with complex supply chains, a mix of methods could work best. Always consider your specific data and business needs when choosing a method.
Q2. How far in advance should I forecast inventory?
Forecasting should ideally cover both the short-term (1–3 months) for immediate needs and long-term (6–12 months) for strategic planning. Short-term forecasts help with stock replenishment, while long-term forecasts align with production or procurement cycles. Regularly update your forecasts based on new data and changing market conditions.
Q3. How do I handle forecasting for new products with no historical data?
For new products, you can estimate demand by analyzing similar products' performance, considering market research, and factoring in promotional activities. Start with a conservative forecast and adjust as actual data becomes available. Using a Delphi Method (getting expert opinions) or relying on market trends can also be helpful for new product forecasting.
Q4. Can I use inventory forecasting for perishable goods?
Yes, inventory forecasting is particularly crucial for perishable goods. For products with a limited shelf life, forecasting methods such as Exponential Smoothing or Moving Average can help adjust to seasonal variations and minimize waste. You should also integrate real-time sales data and closely monitor trends to ensure quick action if demand fluctuates.
Q5. What happens if my inventory forecast is wrong?
An inaccurate forecast can result in either stockouts or overstocking. Stockouts lead to lost sales and customer dissatisfaction, while overstocking ties up cash and incurs unnecessary storage costs. To mitigate this, maintain a safety stock buffer and continually refine your forecasting methods. Regularly review performance metrics like MAPE (Mean Absolute Percentage Error) to assess and improve forecast accuracy.
Q6. How do I improve the accuracy of my inventory forecasts?
Improving accuracy involves:
- Gathering high-quality data: Ensure your sales and inventory data are accurate and up-to-date.
- Adjusting for seasonality: Use seasonal indices to factor in demand fluctuations.
- Regular reviews: Continuously update forecasts based on new data and trends.
- Using advanced forecasting methods: Leverage machine learning or AI-based tools to predict demand more precisely.
Q7. What is the role of safety stock in inventory forecasting?
Safety stock serves as a buffer to protect against unforeseen demand spikes or delays in supply. It’s particularly useful when forecasting methods don’t capture all variables, such as unexpected surges in demand or shipping delays. By maintaining safety stock, you ensure that you can fulfill customer orders even when demand is higher than expected or lead times are longer.
Q8. How do I forecast for seasonal demand?
For seasonal demand, methods like Seasonal Index and Exponential Smoothing can adjust your forecasts to account for known demand fluctuations during specific periods. By analyzing historical data, you can predict peak seasons (e.g., holidays, weather changes) and plan inventory accordingly. Make sure to update your forecasts each year based on actual sales performance from previous seasons.
Q9. What’s the difference between inventory forecasting and replenishment?
Inventory forecasting is the process of predicting future demand for products, which helps determine how much inventory you’ll need at any given time. Inventory replenishment, on the other hand, is the process of restocking products to meet the forecasted demand. Forecasting informs replenishment, ensuring that stock levels are aligned with expected customer demand.
Q10. How do I account for external factors like market trends in forecasting?
External factors such as changes in consumer behavior, economic shifts, or competitor actions should be incorporated into your forecasting models. You can do this by using trend analysis or market research to adjust forecasts based on broader market conditions. Advanced forecasting models like machine learning algorithms can also incorporate external data sources to improve predictions.
Optimize Your Inventory Effortlessly
Receive timely insights and updates to ensure your inventory stays perfectly aligned with demand.
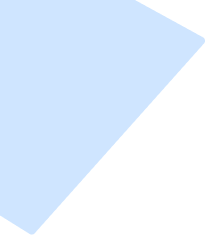
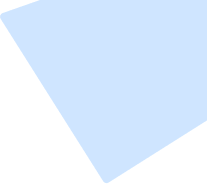

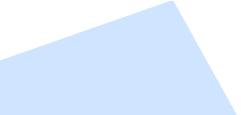