AI Inventory Management: Process, Solution & Benefits

Manual inventory methods can’t keep up with today’s multi-channel, high-velocity supply chains. Fluctuating demand, rising logistics costs, and limited visibility across warehouse and retail locations create inefficiencies that drain revenue. That’s why businesses are turning to AI in inventory management to automate critical tasks like demand forecasting, inventory classification, replenishment planning, and anomaly detection—transforming static systems into intelligent, self-optimizing engines.
Whether you're running an e-commerce store, a global supply chain, or a growing retail brand, the use of AI in inventory management systems can drive higher efficiency, lower costs, and improve customer satisfaction.
In this blog, we’ll explore how AI is used in inventory management, the key benefits it offers, and how you can start leveraging it to gain a competitive edge in today’s data-driven world.
What Is AI Inventory Management?
AI inventory management refers to the use of artificial intelligence technologies—like machine learning, predictive analytics, and automation—to optimize how businesses track, manage, and replenish their inventory. Unlike traditional methods that rely heavily on static rules and manual oversight, AI-driven systems learn from data patterns and make real-time decisions to improve accuracy, efficiency, and responsiveness across the supply chain.
These systems continuously analyze sales data, customer demand, supply chain variables, and even external factors (like weather or economic shifts) to forecast needs and automate stock-related actions. The result? Lower carrying costs, fewer stockouts, faster replenishment, and a more agile operation.
How Is AI Used in Inventory Management?
AI is transforming inventory management by turning reactive systems into predictive and automated workflows. Instead of relying on historical averages or manual input, AI algorithms analyze large volumes of real-time and historical data—such as sales trends, seasonality, supplier lead times, and external factors like weather or promotions—to generate accurate forecasts and inventory decisions.
Here are some of the most common and effective use cases of AI in inventory management.
- Demand Forecasting: Machine learning models predict future product demand based on multi-variable inputs, helping businesses avoid overstocking or stockouts.
- Automated Replenishment: AI-powered systems trigger restocking decisions based on real-time stock levels and forecasted demand, reducing human error.
- Inventory Classification: AI clusters SKUs based on turnover rate, profitability, or seasonality, enabling smarter prioritization and resource allocation (e.g., ABC analysis enhanced by machine learning).
- Anomaly Detection: AI models can flag sudden inventory discrepancies, theft risks, or supply chain delays in real-time, allowing for proactive mitigation.
- Dynamic Safety Stock Setting: Instead of fixed buffers, AI adjusts safety stock levels based on ongoing data inputs like lead time variability or demand volatility.
Real-Life Example:
Zara, a global fashion retailer, uses AI-powered inventory systems to forecast which products are likely to trend in specific regions. Based on in-store sales data, social media signals, and supply chain metrics, the system suggests optimized stock distribution across stores. This has allowed Zara to reduce markdowns and improve sell-through rates—especially for fast-moving seasonal collections.
Benefits of AI-Powered Inventory Management
Implementing AI in your inventory management system doesn’t just add automation—it fundamentally upgrades how your business anticipates, reacts, and scales. Below are the key benefits businesses experience when they adopt AI for inventory management automation:
1. Accurate Demand Forecasting
AI algorithms process sales trends, seasonality, market behavior, and external data (like weather or events) to predict demand with far greater accuracy than traditional methods. This helps reduce overstocking and understocking—both of which eat into profits.
2. Automated Replenishment & Ordering
By setting dynamic reorder points based on real-time stock levels and predicted demand, AI systems can automate purchase orders without human intervention. This reduces manual errors and ensures inventory availability at the right time and place.
3. Optimized Inventory Levels & Reduced Carrying Costs
AI continuously analyzes product movement and storage data to maintain optimal stock levels. This means less capital tied up in slow-moving inventory, reduced warehousing costs, and improved cash flow.
4. Improved Supply Chain Visibility & Responsiveness
AI-powered tools integrate data across suppliers, warehouses, and sales channels to give a unified, real-time view of your inventory. If there’s a delay in shipping or a sudden demand spike, the system can adjust forecasts and reorder logic on the fly.
5. Proactive Risk Management
Machine learning models detect anomalies—like unexpected stockouts, demand spikes, or supplier delays—before they become problems. This allows your team to make faster decisions and reduce disruptions.
How to Manage Inventory with AI: A Practical Approach
Adopting AI for inventory management doesn’t require a complete systems overhaul—but it does require a structured approach. Here’s how businesses can start integrating AI into their inventory workflows and get real, measurable results:
1. Audit Your Current Inventory Systems
Start by evaluating your existing tools, processes, and data infrastructure. Do you have clean, consistent sales and inventory data? Are your systems (ERP, WMS, POS) integrated? AI thrives on data—so your foundation must be solid.
2. Define Clear Inventory Goals
AI models perform best when trained with purpose. Define what you want to improve:
- Reduce stockouts or overstock?
- Automate reordering?
- Forecast seasonal demand more accurately?
Clear KPIs help in selecting the right AI solution and measuring its impact.
3. Choose the Right AI-Powered Inventory Tool
There are purpose-built platforms that embed AI and machine learning into inventory management. Look for solutions that support:
- Real-time data processing
- Predictive analytics
- Automated replenishment logic
- Multi-channel inventory visibility
Examples: NetSuite (Oracle), E2open, Lokad, or custom AI integrations using Python/ML frameworks for advanced teams.
4. Train AI Models with Historical Data
Once implemented, feed the system with historical sales, stock, and supplier performance data. The more data AI can analyze, the more accurate its predictions and recommendations will be.
5. Start Small, Then Scale
Begin with one product category, warehouse, or region. Monitor improvements in forecast accuracy, inventory turns, and fulfillment speed—then scale across your operation.
Final Thoughts: Is AI the Right Fit for Your Inventory Strategy?
AI in inventory management is no longer a futuristic concept—it’s a competitive advantage. Businesses that integrate AI are not just improving stock accuracy; they’re making faster decisions, reducing operational waste, and delivering better customer experiences.
However, AI is not a one-size-fits-all solution. If your business handles high SKU volumes, multi-location warehouses, or faces unpredictable demand patterns, AI inventory management can deliver immediate ROI. But for companies with limited data or simpler inventory needs, starting with analytics-driven forecasting or basic automation may be more practical before scaling into full AI adoption.
Additional FAQs About AI in Inventory Management
Q1. How does AI improve inventory turnover rates?
AI optimizes stock levels by accurately predicting demand and automating replenishment, which reduces excess inventory and increases inventory turnover, leading to faster cash flow.
Q2. Can AI help reduce waste and spoilage in inventory management?
Yes. AI models can forecast product expiration dates and demand fluctuations, enabling businesses—especially in perishable goods—to minimize waste and spoilage through smarter stock rotation and timely reordering.
Q3. What industries benefit the most from AI in inventory management?
Retail, e-commerce, manufacturing, healthcare, and fashion industries benefit significantly due to high SKU variety, complex supply chains, and fluctuating consumer demand.
Q4. How does AI handle supply chain disruptions in inventory management?
AI detects early signs of delays or disruptions by analyzing supplier data and external factors, allowing inventory systems to proactively adjust reorder points or source alternatives.
Q5. What role does data quality play in successful AI inventory management?
High-quality, consistent, and comprehensive data is critical for AI accuracy. Poor data can lead to incorrect forecasts and stock decisions, so cleaning and standardizing data is a crucial first step.
Q6. How long does it take to see results after implementing AI in inventory management?
Results vary based on business size and complexity but many companies start seeing improved forecast accuracy and reduced stock issues within 3-6 months after proper AI model training and system integration.
Optimize Your Inventory Effortlessly
Receive timely insights and updates to ensure your inventory stays perfectly aligned with demand.
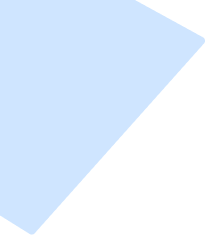
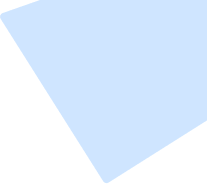

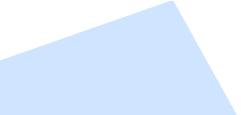