Data-Driven New Season Collection Planning for Fashion Brands
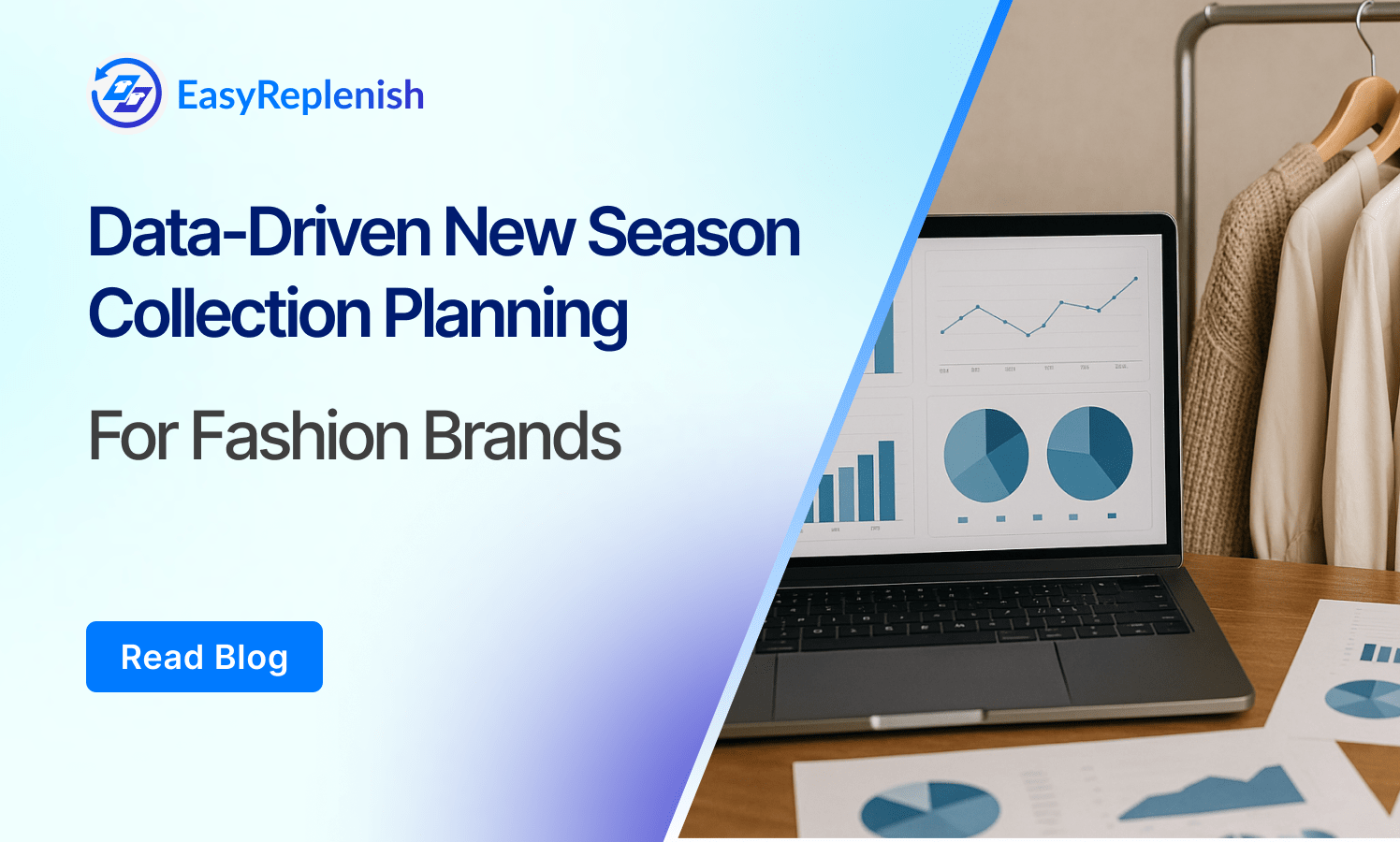
In the fast-paced world of fashion, new season collections are a make-or-break opportunity for brands to captivate their audience, stay ahead of trends, and drive sales. Traditionally, creative intuition and industry experience have guided the planning process. However, as the fashion industry evolves, so too does the need for more sophisticated approaches to collection planning.
Data-driven collection planning is quickly becoming a vital strategy for fashion brands looking to stay competitive and relevant. By harnessing the power of data, brands can not only predict upcoming trends but also align their designs with customer preferences, streamline inventory, and optimize their supply chain. This approach ensures that fashion collections resonate with consumers, reduce waste, and maximize profitability.
In this blog, we’ll explore how fashion brands can leverage data to make informed decisions when planning their new season collections, ensuring they stay on top of trends, meet demand, and keep customers coming back for more
Leveraging Historical Sales Data
When it comes to planning a new season collection, one of the most powerful tools at a fashion brand’s disposal is historical sales data. This treasure trove of information provides invaluable insights into past performance, allowing brands to make informed decisions about which styles, colors, and products to focus on for the upcoming season.
1. Understanding What Worked (and What Didn't)
By diving into past sales figures, brands can easily identify which products had the highest demand. For example, if a specific outerwear style or fabric performed exceptionally well during last winter, this could indicate a trend that’s likely to continue. It’s not just about which items sold best but also about understanding why they resonated with customers. Was it the fabric, the fit, or the color?
Conversely, underperforming products also provide essential data. If a particular silhouette or material didn’t sell as expected, it’s a clear signal for brands to adjust or avoid similar choices in future collections.
2. Spotting Seasonal and Cyclical Trends
Fashion is often driven by seasonal demand, and historical sales data allows brands to pinpoint these cyclical buying patterns. For instance, many brands experience predictable surges in sales during peak shopping seasons such as holiday sales or spring/summer collections. Recognizing these trends in advance means that brands can plan their collections to meet demand at exactly the right time.
3. Optimizing Product Availability
With a clear picture of past demand, fashion brands can also optimize inventory for the upcoming season. By forecasting which products are likely to perform well, they can ensure they have the right quantity of stock on hand. This reduces the risk of both understocking (missing out on sales) and overstocking (leading to excess inventory or markdowns).
4. Multi-Channel Insights
Don’t limit the analysis to just one sales channel. By integrating data from various touchpoints—such as eCommerce platforms, brick-and-mortar stores, and third-party retailers—brands can gain a more holistic view of their sales performance. This broader perspective provides a more accurate understanding of customer preferences across different demographics, regions, and shopping behaviors, helping to shape the new season collection more effectively.
Customer Insights and Market Research
While historical sales data provides a solid foundation, understanding what customers want in real-time is crucial for planning a successful new season collection. This is where customer insights and market research come into play. By tapping into these sources, fashion brands can gain a deeper understanding of consumer desires, emerging trends, and unmet needs.
1. Gathering Feedback Directly from Customers
One of the most effective ways to gather insights is through direct customer feedback. Surveys, reviews, and focus groups provide valuable data on customer preferences, pain points, and opinions on existing products. For instance, if a brand notices consistent feedback that their sizing runs small, they can adjust the new collection to offer better fits or extended sizing options.
Furthermore, brands can track customer satisfaction levels and product ratings to gauge how well their previous collections performed. This data can reveal if certain designs were particularly popular or if there were specific features that customers loved (or disliked).
2. Social Listening: Tapping Into Emerging Trends
Social media is a goldmine for fashion insights, offering an unfiltered view of what consumers are talking about, sharing, and posting. By using social listening tools, fashion brands can monitor discussions on platforms like Instagram, Twitter, Pinterest, and TikTok to identify emerging trends in real-time.
For example, if influencers or everyday users start showcasing a particular style, fabric, or color, it’s a signal that this trend may soon gain mainstream traction. Social listening can also reveal what’s not working for consumers, such as specific trends or brands that are falling out of favor. This allows fashion brands to act quickly and adapt their upcoming collections to align with consumer sentiment.
3. Competitive Analysis
Market research also involves keeping a close eye on what competitors are doing. By studying successful collections from industry leaders, fashion brands can gain insight into popular styles, pricing strategies, and marketing tactics. However, it’s not just about imitation. It’s about differentiation—finding ways to offer something new, innovative, or uniquely tailored to your target audience.
Additionally, analyzing competitors’ customer reviews can reveal gaps in the market. Perhaps there’s a growing demand for more sustainable fashion options, or a specific product category is underrepresented in the market. By identifying these gaps, brands can create collections that meet consumer demands and capture market share.
4. Predicting Consumer Behavior with Data Analytics
Leveraging data analytics tools can further enhance market research by allowing brands to predict future trends. By analyzing large datasets, including demographic information, purchasing history, and browsing behavior, brands can gain a better understanding of how different segments of consumers are likely to behave in the future.
For example, AI-powered tools can analyze consumer behavior and recommend which styles, colors, or types of garments will perform best with specific groups—be it based on age, location, or even interests. This predictive modeling gives fashion brands the power to tailor their collections more precisely, ensuring they meet the specific needs of their target audience.
Using Predictive Analytics for Trend Forecasting
Trend forecasting has always been a cornerstone of fashion collection planning. However, traditional methods of forecasting—relying on intuition, historical trends, and industry expertise—are increasingly being complemented by predictive analytics. Predictive analytics leverages advanced algorithms and data models to forecast trends with greater accuracy, helping brands make data-driven decisions months before the new season hits.
1. How Predictive Analytics Works
By analyzing a variety of data sources—ranging from historical sales and consumer behavior to social media signals—predictive analytics tools can identify patterns and emerging trends that might otherwise go unnoticed. For instance, AI-powered models can track product popularity across regions and monitor fashion influencers, identifying subtle shifts in consumer preferences that indicate the next big thing.
Predictive analytics can also take into account external factors, such as economic shifts, global events, and cultural movements, all of which can influence consumer behavior and fashion trends. By incorporating these variables, brands can stay ahead of the curve and produce collections that are not only in line with current trends but also positioned for future success.
2. AI and Machine Learning in Trend Prediction
AI and machine learning further enhance the power of predictive analytics by processing vast amounts of data faster and more accurately than human analysts ever could. These technologies continuously learn and improve their predictions as more data is fed into them, allowing brands to refine their forecasts and make adjustments in real-time.
For example, machine learning models might predict that a certain shade of green will be popular in the upcoming season based on consumer sentiment analysis and historical data. With this insight, fashion brands can incorporate that specific shade into their new collection, knowing it will resonate with their target audience.
3. Benefits of Predictive Analytics
- Faster Trend Identification: Brands can spot trends early, ensuring they’re among the first to capitalize on them.
- Minimized Risk: Predictive models help reduce the uncertainty in trend forecasting, lowering the chances of creating collections that miss the mark.
- Optimized Product Mix: Brands can refine their collection offerings, ensuring the right styles, colors, and fabrics are included based on predicted demand.
Inventory and Supply Chain Optimization
Once a new season’s collection is planned, ensuring that products are available at the right time and in the right quantities is crucial. Inventory and supply chain optimization relies heavily on data to align production with demand, minimizing the risk of overstocking or stockouts while maximizing profitability.
1. Forecasting Demand to Balance Stock Levels
Accurate demand forecasting is at the core of inventory optimization. By analyzing sales data from previous seasons, alongside real-time customer preferences, fashion brands can predict how much of each product will be needed. Predicting demand accurately means that brands can plan their production and stock levels to ensure they can meet consumer needs without producing excess inventory.
For example, if data indicates that a particular style of jacket has been a consistent bestseller during autumn months, brands can prioritize manufacturing that style in larger quantities to meet expected demand. Conversely, products with lower demand can be produced in smaller batches or not at all, reducing the risk of overproduction and markdowns.
2. Using Technology for Real-Time Supply Chain Management
Real-time supply chain management is essential for efficient inventory optimization. By implementing advanced inventory management systems and supply chain tracking technologies, fashion brands can gain real-time visibility into inventory levels, stock movement, and production schedules. This helps ensure that each product is delivered at the right time, avoiding delays or disruptions that could impact sales.
Additionally, by integrating AI-powered systems that monitor external factors such as global shipping trends, weather patterns, and geopolitical events, brands can anticipate and adapt to potential supply chain challenges. This real-time data enables brands to make swift decisions and adjust their supply chain processes to keep things running smoothly.
3. Minimizing Waste and Maximizing Sustainability
Data-driven inventory management isn’t just about improving profits—it’s also about making more sustainable choices. By accurately forecasting demand and optimizing stock levels, brands can reduce overproduction and minimize waste. This not only saves resources but also contributes to more sustainable business practices in an industry that is often criticized for its environmental impact.
Furthermore, brands can use sustainable materials or production processes based on data insights, ensuring that their supply chain is both efficient and eco-friendly. With increasing consumer demand for sustainable fashion, brands that leverage data to reduce waste and optimize their supply chain will build stronger reputations and gain a competitive edge.
Collaborating with Designers and Data Teams
Incorporating data into collection planning doesn’t mean replacing the creative process—it means enhancing it. The most successful fashion collections are the result of collaboration between the creative design teams and data-driven analysts. By blending the artistic vision of designers with the insights provided by data, brands can create collections that are both stylish and strategically aligned with customer demand.
1. Bridging the Gap Between Creativity and Data
Designers bring a wealth of experience and intuition to the table, but data adds a layer of empirical evidence that helps guide those instincts. Data can reveal what styles, colors, and fits are likely to perform well, while designers can use this insight to create designs that feel fresh, unique, and aligned with market demand.
For example, designers might use data insights to adjust the proportions of a garment, knowing that certain fits are trending or performing better in specific regions. Alternatively, data might suggest that consumers are gravitating toward more sustainable fabrics, which can inspire designers to explore eco-friendly materials without compromising on design or quality.
2. Iterative Collaboration for Better Outcomes
Rather than relying on a top-down approach, collaboration between the data and design teams should be iterative. Designers can create prototypes and receive feedback from data analysts based on customer preferences, sales patterns, and predictive analytics. This cycle of continuous feedback and refinement allows the collection to evolve with both creative and data-driven input.
This collaborative process also enables brands to be more agile, making adjustments to designs or inventory plans if new insights emerge during the development stage. With the right communication and shared goals, the combination of creative passion and data-driven insights ensures that collections resonate with consumers while remaining profitable and relevant.
3. Building a Data-Driven Culture
Creating a data-driven culture within the fashion brand is key to success. By fostering an environment where both designers and data analysts can work together seamlessly, brands can align their teams around a shared vision—one that uses data to inform creative decisions rather than stifling creativity. Training designers to understand and utilize basic data insights can further strengthen this collaboration, allowing for more informed and dynamic collections.
Examples and Case Studies
Real-world examples of brands successfully integrating data into their collection planning process can provide a clearer picture of how these strategies work in practice. Let’s look at a few fashion companies that have leveraged data-driven insights to create highly successful collections.
Case Study 1: Zara – Real-Time Data for Rapid Response
Zara, one of the leading fast fashion brands, is famous for its agile supply chain and rapid response to consumer demand. The company uses real-time data from its stores, online platforms, and customer interactions to continually monitor buying patterns. This allows Zara to design, manufacture, and deliver new styles in as little as two weeks, ensuring they meet emerging trends as they unfold. Their ability to quickly adapt to consumer feedback through data has helped them maintain relevance in the competitive fashion industry.
Case Study 2: H&M – Data-Driven Sustainability
H&M is another fashion brand that has leveraged data to enhance both its trend forecasting and sustainability efforts. By using data analytics, H&M has been able to predict which styles are likely to sell well and which are not, reducing the amount of unsold inventory and minimizing waste. In addition, data has allowed H&M to better forecast the demand for sustainable materials and eco-friendly products, which aligns with their commitment to sustainability. Their data-driven approach ensures that both customer preferences and environmental concerns are addressed in every new collection.
Case Study 3: Stitch Fix – Personalized, Data-Driven Curation
Stitch Fix, an online personal styling service, uses AI and data analytics to tailor clothing selections for each customer based on their style preferences, size, and purchase history. By analyzing vast amounts of data on customer behavior and fashion trends, Stitch Fix’s stylists can curate personalized collections for each customer, resulting in a higher rate of customer satisfaction and increased sales. This highly personalized approach demonstrates how data can be used not just for trend forecasting but for creating individualized collections that meet the needs of every consumer.
Lessons Learned from Case Studies
These examples show that data-driven collection planning can significantly improve efficiency, reduce waste, and boost profitability. Brands that embrace data not only stay ahead of trends but also deliver products that customers genuinely want, ensuring higher sales and a stronger brand reputation. The key takeaway is that when fashion companies combine creativity with data-driven insights, they can create collections that are both fashionable and strategically sound.
Conclusion
Data-driven collection planning is no longer a luxury—it’s a necessity for fashion brands looking to stay competitive and relevant in an ever-evolving industry. By leveraging historical sales data, customer insights, predictive analytics, and real-time supply chain management, brands can ensure that their new season collections meet customer demand, reduce waste, and maximize profitability.
The key to success lies in collaborating across teams—designers, data analysts, and supply chain managers—to create collections that are not only visually appealing but also strategically sound. Embracing technology and data tools allows brands to respond quickly to emerging trends, forecast demand with accuracy, and produce products that resonate with their audience.
As the fashion industry continues to embrace data-driven decision-making, brands that integrate data insights into their creative processes will be better equipped to thrive in the future. Ultimately, a data-driven approach empowers brands to make smarter decisions, create products that customers love, and maintain a sustainable and profitable business model.
Frequently Asked Question
Q1. How can small fashion brands implement data-driven collection planning?
Small fashion brands can start by focusing on accessible data sources, such as customer feedback from online reviews and social media insights. Even without large-scale data infrastructure, brands can use affordable tools like Google Analytics, social listening platforms, and basic sales tracking to understand their customers’ preferences. Partnering with third-party data providers or utilizing basic predictive analytics tools can help small brands forecast trends and optimize their collections for maximum impact.
Q2. What role does artificial intelligence play in data-driven collection planning?
Artificial intelligence (AI) plays a significant role by analyzing large datasets more efficiently than human analysts. AI can detect emerging trends, predict customer behavior, and help brands optimize inventory levels. Machine learning models continuously improve their predictions, allowing brands to stay ahead of the curve and respond quickly to changes in consumer preferences. AI tools can also personalize collections based on customer data, enhancing the shopping experience and increasing sales.
Q3. How can fashion brands ensure sustainability while using data for collection planning?
Data-driven collection planning can help fashion brands become more sustainable by accurately forecasting demand, reducing overproduction, and minimizing waste. By using data insights to predict which items will sell and which won't, brands can avoid creating excess inventory that ends up in landfills. Additionally, data can help brands identify customer demand for sustainable materials and eco-friendly practices, guiding the design and production of more sustainable collections.
Q4. Can predictive analytics help fashion brands avoid overstocking or understocking?
Yes, predictive analytics is a powerful tool for inventory optimization. By analyzing past sales data, market trends, and external factors, predictive models help brands forecast the demand for each product more accurately. This allows brands to align their production schedules with expected demand, reducing the risk of both overstocking (leading to excess inventory and markdowns) and understocking (resulting in missed sales opportunities). With better demand forecasting, brands can maintain optimal inventory levels and improve overall profitability.
Optimize Your Inventory Effortlessly
Receive timely insights and updates to ensure your inventory stays perfectly aligned with demand.
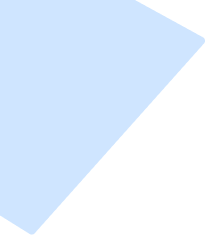
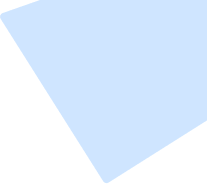

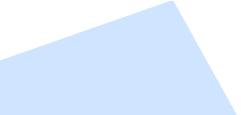