E-Commerce Demand Forecasting: Techniques, Tools & KPIs (2025)
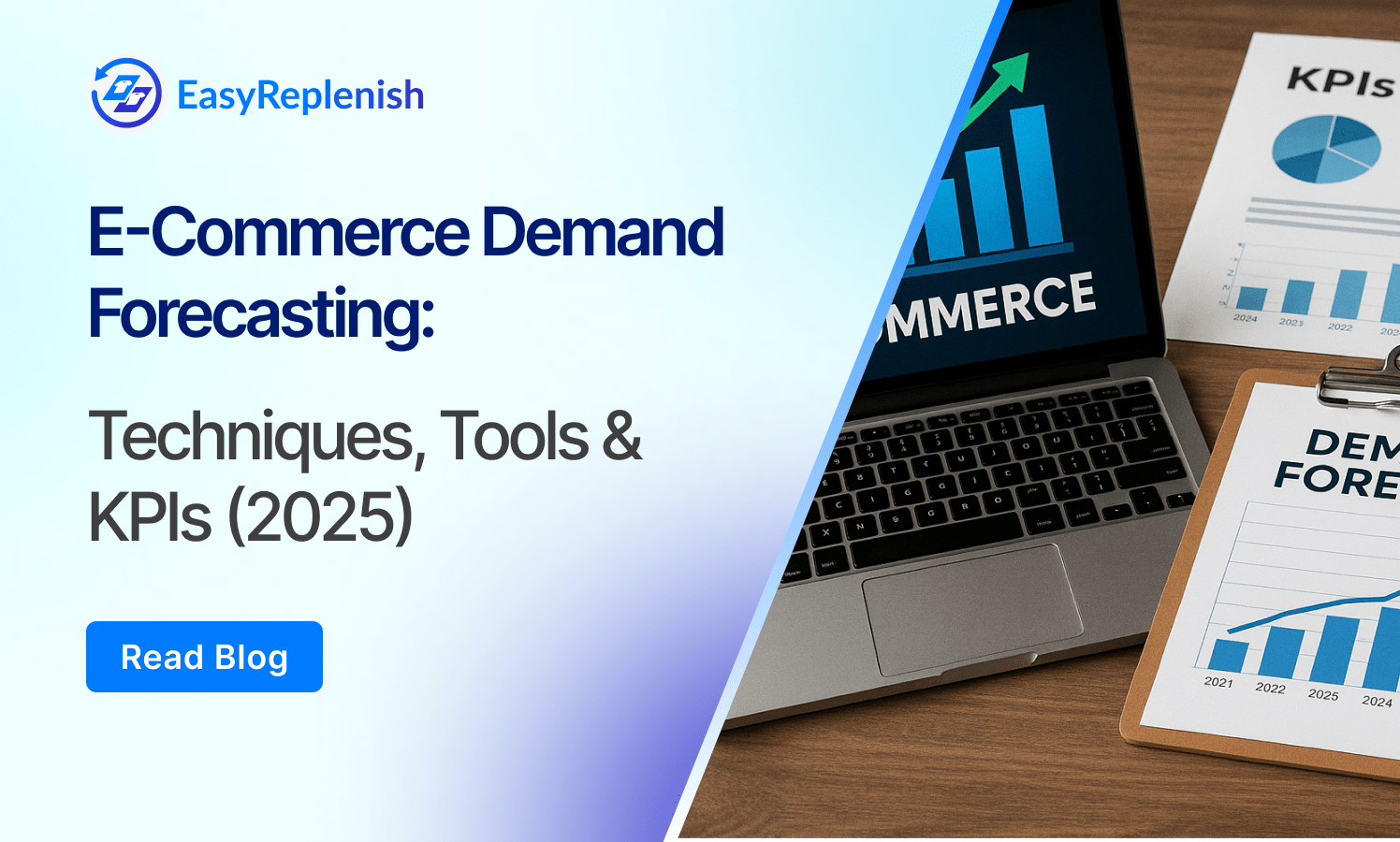
What is eCommerce Demand Forecasting?
eCommerce demand forecasting is the process of predicting future customer demand for products sold through online channels. It involves analyzing historical sales data, market trends, seasonality, and external factors to estimate how much inventory a business will need in the coming days, weeks, or months.
In a digital-first retail landscape, demand forecasting in eCommerce has evolved from gut-feel decisions to data-driven strategies. Today, with the help of machine learning and AI, brands can forecast demand more accurately than ever before—allowing them to reduce inventory costs, avoid stockouts, and deliver better customer experiences.
Whether you’re managing a single Shopify store or overseeing a multi-channel retail operation, having a reliable eCommerce demand forecasting process can make or break your profitability. It ensures that your supply chain, marketing campaigns, and cash flow are aligned with actual customer behavior.
The Business Impact of Accurate Demand Forecasting
Accurate demand forecasting is not just a supply chain function—it’s a strategic growth driver for eCommerce businesses. By predicting demand with precision, you can make smarter decisions across operations, finance, procurement, and customer experience.
Here’s how accurate eCommerce demand forecasting directly impacts your business:
1. Reduces Stockouts and Lost Sales
When you forecast demand correctly, you’re less likely to run out of best-selling SKUs. This means fewer missed sales opportunities and improved customer satisfaction.
2. Prevents Overstock and Excess Inventory
Overestimating demand can tie up cash in unsold inventory and increase warehousing costs. Forecasting allows you to optimize purchasing and minimize waste.
3. Improves Marketing and Promotion Planning
Forecasting helps you understand when customers are most likely to buy. You can align ad spend and campaigns with high-demand periods to boost ROI.
4. Enables Agile Supply Chain Operations
From supplier lead times to reordering cycles, forecasting gives operations teams the visibility they need to make informed, timely decisions.
5. Supports Scalable Growth
With automated demand forecasting systems, you can scale operations confidently—adding new products, channels, or regions without losing control over inventory.
Core Types of eCommerce Demand Forecasting
Different eCommerce businesses require different forecasting models depending on product types, data availability, and sales patterns. Understanding the types of demand forecasting in eCommerce helps you choose the right model for your growth stage and operational complexity.
1. Qualitative Forecasting
Qualitative forecasting relies on expert opinions, market research, and intuition rather than historical sales data. This approach is ideal for:
- New product launches with no prior sales data
- Niche markets with highly unpredictable demand
- Short-term forecasting driven by promotions, influencer campaigns, or PR spikes
Common methods include:
- Executive opinions
- Delphi method (expert consensus)
- Customer surveys and focus groups
Use Case: A DTC skincare brand launching a new anti-aging serum may use influencer insights and trend data to project initial demand.
2. Quantitative Forecasting
This data-driven approach uses past sales and operational metrics to predict future demand. It’s the most common method used in scaling eCommerce brands.
Popular quantitative forecasting models include:
- Time series forecasting (ARIMA, exponential smoothing)
- Moving averages
- Machine learning algorithms trained on sales history
Use Case: A fashion retailer analyzes three years of Black Friday sales data to forecast demand for upcoming seasonal SKUs.
3. Causal Forecasting
Causal models go beyond past sales to factor in external influences such as pricing, advertising, macroeconomic shifts, and competitor actions.
This model is useful when:
- Promotional campaigns or price discounts heavily influence demand
- Seasonality and holidays create temporary demand spikes
- Market behavior changes due to social or economic trends
Use Case: An electronics brand uses causal forecasting to measure how a 15% discount during Cyber Monday impacts smartphone demand compared to regular pricing.
4. Hybrid Forecasting Models
Hybrid models combine qualitative, quantitative, and causal techniques to improve forecast accuracy. Many AI-powered demand forecasting tools use hybrid forecasting algorithms to adapt to changing data environments in real-time.
Use Case: A multichannel brand uses historical sales data (quantitative), influencer-driven trends (qualitative), and PPC ad spend (causal) to create one unified demand forecast.
Key Inputs for Accurate Demand Forecasting
The quality of your demand forecast is directly tied to the quality of input data. To build reliable and actionable forecasts, eCommerce businesses must centralize and clean a variety of structured and unstructured data sources.
Here are the most critical demand forecasting inputs for eCommerce:
1. Historical Sales Data
This is the backbone of quantitative forecasting. Analyze:
- Daily, weekly, and monthly sales volumes
- Order frequency by SKU
- Sales velocity across channels
Tip: Identify anomalies due to stockouts, flash sales, or marketing events so your model doesn’t over- or under-predict.
2. Seasonality and Event-Based Trends
Track how demand fluctuates during:
- Holidays (e.g., Christmas, Diwali, Black Friday)
- Weather or seasonal cycles (e.g., winter jackets vs. summer wear)
- Major events (e.g., product launches or promotions)
AI models trained on seasonal patterns improve accuracy by anticipating demand spikes or dips.
3. Marketing Campaigns and Promotions
Forecasts must factor in traffic spikes from:
- Email or SMS campaigns
- Influencer promotions
- Google and Meta ad spend
- Flash sales and coupons
Even small marketing changes can have a huge impact on demand.
4. Product Availability and Inventory Levels
Out-of-stock periods can mislead forecasting models. Always track:
- Days of stockout
- Backorder rates
- Inventory turnover
- Replenishment lead times
Pro tip: Sync forecasting models with real-time inventory management systems for dynamic predictions.
5. Customer Behavior and Site Analytics
Behavioral insights from tools like Google Analytics or Hotjar can indicate upcoming demand shifts:
- Product page visits
- Add-to-cart ratios
- Wishlist or waitlist activity
- Exit rates during high demand
6. Macroeconomic and External Factors
Don’t ignore the big picture. Macroeconomic signals like inflation, geopolitical events, and consumer confidence influence eCommerce demand—especially for high-ticket or discretionary goods.
Methods and Techniques Used in eCommerce Demand Forecasting
Choosing the right demand forecasting method is key to building accurate and scalable predictions. While traditional models still play a role, modern eCommerce businesses increasingly rely on AI and machine learning to handle large datasets and dynamic environments.
Here’s a breakdown of the most effective demand forecasting techniques for eCommerce:
1. Time Series Forecasting
Time series models use historical data points indexed by time (daily, weekly, monthly) to predict future demand patterns. Ideal for SKUs with stable, repeatable sales cycles.
Popular methods:
- ARIMA (Auto-Regressive Integrated Moving Average): Best for trend + seasonality
- Exponential Smoothing (ETS): Reacts to recent trends more heavily
- Holt-Winters Method: Adds seasonality to exponential smoothing
Use this when: You have stable historical data with clear seasonality or recurring trends.
2. Machine Learning & AI-Based Forecasting
ML and AI models are highly adaptive and can detect complex patterns across multiple variables. These algorithms train on vast datasets and update predictions as new data becomes available.
Top algorithms:
- XGBoost / Random Forest: Good for structured tabular data and feature importance
- LSTM (Long Short-Term Memory): Neural networks built for sequential data (great for time-based trends)
- Facebook Prophet: Designed for business time series forecasting with seasonality and holidays
Use this when: You want a scalable, automated system that adjusts to new trends, promotions, and demand volatility.
3. Moving Averages and Trend Analysis
Simple yet effective, moving averages smooth out fluctuations to show trends over time. Often used as a baseline forecast for demand planning.
Types:
- Simple Moving Average (SMA)
- Weighted Moving Average (WMA)
Use this when: You need a lightweight forecast or want to benchmark against more advanced models.
4. Regression Analysis
Regression models help identify and quantify relationships between sales and influencing factors like ad spend, price changes, or weather.
- Linear regression is used for straightforward demand influencers
- Multiple regression adds complexity by factoring in several variables at once
Use this when: You want to analyze how specific factors (e.g., marketing budget) impact demand.
5. Ensemble Forecasting and Hybrid Models
Advanced businesses combine multiple forecasting techniques to improve accuracy. For example, pairing ARIMA (to capture seasonality) with XGBoost (to include promotional data).
Use this when: Your product mix is large and demand drivers vary widely by SKU or channel.
Real-World Use Cases and Industry Examples
1. Fashion & Apparel: Managing Seasonal Surges
In the fashion industry, demand forecasting is primarily influenced by seasonality—the changes in customer preferences tied to weather, holidays, and fashion trends. A major challenge for fashion brands is the rapid shift in demand, driven by unpredictable trends, influencer marketing, and seasonal collections.
Example:
A global fashion retailer needs to predict demand for its summer collection well in advance, taking into account past sales patterns, trend forecasts, and seasonal marketing campaigns.
The brand uses time series models combined with causal factors like influencer endorsements and social media trends to predict demand across various regions. For instance, forecasting sales for swimwear in California might be quite different from sales in New York due to regional seasonal variations.
How it works in practice:
The retailer uses ARIMA models to analyze past sales, factoring in historical summer demand over several years.
Additionally, they incorporate real-time data from platforms like Instagram and TikTok, analyzing which items are receiving the most engagement. They also adjust the forecast based on historical sales during key events like Black Friday and Cyber Monday to predict demand surges or drops.
Result: The fashion brand can place bulk orders for popular items ahead of time, reduce overstock of less popular designs, and ensure that their seasonal collections arrive at the right time without excess inventory costs.
2. Consumer Electronics: Forecasting Short Product Lifecycles
In the consumer electronics industry, demand forecasting is especially challenging due to rapid technological advancements and short product lifecycles. Forecasting demand accurately for tech products like smartphones or smartwatches requires considering both historical sales and external data points such as market sentiment, competitor releases, and consumer reviews.
Example:
A major smartphone brand is preparing for the release of a new model. Instead of solely relying on historical sales, they leverage machine learning models to predict consumer interest based on a mix of social media chatter, competitor releases, and pre-order data. The forecast also takes into account supply chain lead times for production and distribution to avoid stockouts during launch.
How it works in practice:
The brand uses LSTM (Long Short-Term Memory) neural networks to analyze data from previous product launches, consumer behavior signals, and even market shifts like economic downturns that could impact buying behavior. They track product pre-orders and monitor online reviews and tech blog mentions to predict post-launch demand.
Result: With a highly accurate forecast, the brand can optimize production runs and ensure product availability across multiple regions. They also avoid overproduction, preventing the loss of margin due to unsold inventory or the risk of running out of stock.
3. FMCG (Fast-Moving Consumer Goods): Predicting High-Volume SKU Sales
FMCG brands sell products with high turnover, meaning demand forecasting plays a critical role in ensuring there is just enough stock to meet customer demand. Stockouts can cause loss of revenue, while excess inventory leads to increased storage and waste. Forecasting for FMCG involves using historical sales data, market trends, weather patterns, and even political changes to predict demand for perishable or fast-moving items like beverages, snacks, and personal care products.
Example:
A large beverage company forecasts demand for its soda line during the summer months. Since consumer demand for cold beverages spikes in warm weather, the company needs to adjust its inventory levels well before the hot season begins. The forecast also accounts for weather patterns, promotions, and retailer demand signals.
How it works in practice:
The brand uses time series models combined with weather data and real-time POS (point-of-sale) data from retailers. They forecast demand based on historical sales for the same period in previous years, while factoring in weather predictions (hotter-than-usual summers lead to higher demand for refreshing drinks).
Result: The brand can increase production and distribute inventory to high-demand areas in advance, ensuring that they don’t face shortages during peak demand periods, while also reducing waste in regions where demand is lower.
4. Beauty & Skincare: Planning Promotions and Influencer Campaigns
The beauty and skincare industry often experiences volatile demand due to the influence of marketing events, influencer endorsements, and seasonal trends. Accurate demand forecasting is essential to capitalize on short-lived spikes in interest, especially when planning around product launches, limited-time promotions, or viral campaigns.
Example:
A luxury skincare brand forecasts demand for a newly launched anti-aging serum by incorporating influencer marketing data, influencer reviews, and customer sentiment analysis. Since influencer-driven marketing can generate rapid interest, the company needs to predict how many units they need in stock post-launch and ensure they can meet demand during key moments like influencer partnerships or YouTube tutorials.
How it works in practice:
Using causal forecasting, the brand analyzes data from influencer campaigns, social media mentions, and prior product launches to predict future demand. They combine this with quantitative data such as historical sales of similar products, adjusting the forecast for seasonal changes and key promotions.
Result: The brand can plan production and distribution schedules accordingly, ensuring they don’t miss out on sales due to a lack of inventory while keeping excess stock to a minimum.
Forecasting for New Product Launches
Launching a new product presents unique challenges in demand forecasting. The primary hurdle is the lack of historical sales data, making it difficult to predict demand with accuracy. However, effective forecasting for new products is critical to avoid stockouts, optimize inventory levels, and align production schedules with expected demand.
1. Challenges with New Product Forecasting
New product forecasting often carries high risks because the absence of sales history makes it harder to establish accurate demand predictions. Additional external factors such as consumer trends, market competition, and media buzz also play significant roles in shaping demand for new products.
The key challenges include:
- Absence of historical sales data: Traditional forecasting models often rely on past sales data, which is unavailable for new products.
- Market uncertainty: New products can face unknown barriers or demand fluctuations that cannot be foreseen based on historical data alone.
- Demand volatility: Influencer campaigns, promotional efforts, and media attention can generate unpredictable demand surges, adding another layer of complexity to forecasting.
Methods for Accurate New Product Forecasting
To tackle the challenges of forecasting new products, businesses employ several methods that can improve the accuracy of predictions, even when historical data is limited:
1. Leverage Similar Product Data (Analogous Forecasting)
In the absence of data for the new product, businesses can utilize demand forecasts from similar or related products that target the same customer base. By analyzing products with comparable features, categories, or consumer segments, businesses can estimate potential demand for the new product.
2. Incorporate Market Research and Consumer Sentiment
Market research, including customer surveys, focus groups, and consumer sentiment analysis, plays a critical role in forecasting demand for new products. This data can help gauge consumer interest, buying intent, and market readiness, which provides valuable insights when estimating initial demand.
3. Utilize Influencer and Social Media Data
Social media platforms and influencer marketing can significantly influence demand for new products, especially in industries like fashion, beauty, and technology. Monitoring social media conversations, mentions, and engagement metrics can offer early indicators of product interest and potential demand spikes.
4. Machine Learning Models and A/B Testing
Machine learning (ML) models can help predict demand by analyzing patterns from previous product launches or consumer behaviors. Additionally, conducting A/B testing prior to the product launch allows businesses to collect valuable real-time data on customer preferences, which can further refine demand predictions.
Overcoming Common Forecasting Challenges
Demand forecasting in eCommerce comes with its own set of challenges. These obstacles can hinder the ability to make accurate predictions, resulting in either stockouts or excess inventory. Addressing these challenges is essential to improving forecasting accuracy and overall business performance.
1. Data Quality Issues
Challenge: Data quality is one of the most significant barriers to accurate demand forecasting. Inconsistent or incomplete data can lead to inaccurate predictions, causing businesses to overstock or run out of critical products.
Solution: Businesses need to implement robust data cleaning and validation processes. This involves ensuring that all data is complete, accurate, and properly formatted before using it for forecasting. Additionally, integrating data from multiple sources, including sales, inventory, and external factors like weather or market trends, enhances forecasting accuracy.
2. Demand Volatility and Unpredictability
Challenge: External factors, such as economic changes, seasonal variations, and unforeseen global events (e.g., pandemics or supply chain disruptions), can introduce demand volatility, making it difficult to forecast with accuracy.
Solution: To handle this unpredictability, businesses can use scenario planning, where multiple forecasts are developed to account for different potential future scenarios (e.g., high demand, low demand, or stable demand). Real-time analytics can also be used to adjust forecasts dynamically, ensuring that businesses stay responsive to changing conditions.
3. Over-Reliance on Historical Data
Challenge: Historical sales data is essential for demand forecasting, but over-reliance on it can lead to inaccurate predictions, especially in fast-moving industries or when introducing new products. Consumer behavior and market conditions change over time, and past data may not always reflect current trends.
Solution: To mitigate this issue, businesses should incorporate additional forecasting methods, such as causal forecasting and machine learning models, which account for external factors like marketing campaigns, competitor activity, and consumer sentiment. These approaches can help businesses predict demand more effectively, even in the absence of perfect historical data.
4. Inaccurate Lead Times and Supply Chain Constraints
Challenge: Long and variable lead times in production or shipping can cause inventory misalignments, leading to stockouts or excess inventory. Supply chain disruptions, including delays in raw material sourcing or transportation, can further complicate demand forecasting.
Solution: Businesses should synchronize their demand forecasts with their suppliers and supply chain teams to ensure realistic lead times are factored into predictions. Using supply chain visibility tools helps identify potential bottlenecks early, allowing businesses to adjust their forecasts and inventory levels accordingly.
5. Limited Visibility into Consumer Behavior
Challenge: Predicting consumer behavior is inherently difficult due to the dynamic nature of buyer preferences, changing trends, and external influences. Demand can fluctuate based on evolving consumer sentiment, marketing efforts, or sudden shifts in the market.
Solution: Businesses can use behavioral analytics to track customer interactions, monitor website activity, and analyze social media discussions. Leveraging sentiment analysis tools can provide real-time insights into consumer attitudes toward products, which can be used to adjust demand forecasts. Additionally, using AI-based models that detect emerging trends can help businesses better anticipate changes in consumer demand.
Choosing the Right Tools & Platforms
When it comes to demand forecasting, selecting the right tools and platforms is crucial to the success of your forecasting strategy. The right technology can automate the process, improve accuracy, and provide real-time insights, enabling your business to optimize inventory levels, reduce costs, and increase profitability. Here's a breakdown of how to choose the right tools for your needs.
Factors to Consider
- Data Integration Capabilities
Your forecasting tool should seamlessly integrate with existing systems such as CRM, ERP, inventory management, and sales platforms. This ensures that data flows smoothly across your business and is available for accurate demand predictions. - Forecasting Methodology Support
Different tools support different forecasting methodologies. Ensure the platform can handle time-series forecasting, machine learning models, and causal forecasting, depending on the complexity of your needs. You may also want tools that can support scenario planning and demand sensing. - Real-time Data Processing
For accurate demand forecasting, real-time data analysis is essential. Choose platforms that provide real-time analytics, allowing you to adjust forecasts quickly based on changing market conditions, consumer behavior, and supply chain disruptions. - User-Friendliness
Look for tools that are easy to use, with an intuitive interface that enables both technical and non-technical teams to interact with the software. This ensures broader adoption across departments and helps streamline decision-making. - Scalability
As your business grows, so too should your forecasting capabilities. Choose tools that can scale with your business needs, handling increasing amounts of data, new product lines, and more complex forecasting requirements.
Top Tools for eCommerce Demand Forecasting
- Easyreplenish: A popular tool that uses machine learning to optimize inventory and demand forecasts. It’s great for eCommerce businesses with diverse product offerings.
- SAP Integrated Business Planning: A comprehensive supply chain management platform that offers advanced demand forecasting, planning, and optimization tools.
- Oracle Demand Management Cloud: Provides robust forecasting and planning features, integrating with ERP and inventory systems to ensure demand accuracy.
- Zoho Inventory: A scalable solution for businesses of all sizes, combining inventory management with powerful demand forecasting tools.
KPIs and Metrics to Track Forecast Accuracy
Tracking the right Key Performance Indicators (KPIs) is crucial for evaluating the effectiveness of your demand forecasting efforts. By monitoring these metrics, businesses can identify areas of improvement, adjust strategies, and continually optimize forecasting accuracy.
Key KPIs to Measure Forecast Accuracy
- Forecast Error (FE) Forecast error measures the difference between predicted demand and actual demand. By analyzing forecast error, businesses can evaluate the overall accuracy of their forecasts and determine the level of inaccuracy. A smaller forecast error indicates better performance, meaning forecasts are closely aligned with actual demand.
- Mean Absolute Percentage Error (MAPE) MAPE expresses forecast accuracy as a percentage difference between forecasted and actual demand. A lower MAPE signifies better forecasting accuracy. This metric is commonly used to compare the performance of different forecasting models or techniques, allowing businesses to determine which methods yield the best results.
- Root Mean Squared Error (RMSE) RMSE measures the magnitude of forecast error by averaging the squared errors and then taking the square root. It gives more weight to larger errors, making it useful when businesses want to penalize large forecasting mistakes. A lower RMSE indicates more accurate predictions and better alignment with actual demand.
- Stockouts and Overstock Rates Stockouts (when demand exceeds supply) and overstocking (when supply exceeds demand) are both direct outcomes of forecasting errors. By tracking these rates, businesses can assess how well their forecasts align with actual demand. Reducing both stockouts and overstocking leads to more efficient inventory management and fewer missed sales or excess inventory costs.
- Forecast Bias Forecast bias helps businesses understand if their forecasts are consistently overestimating or underestimating demand. If a forecast consistently predicts demand too high or too low, it signals a potential problem in the forecasting model or its underlying data inputs. A balanced forecast should have minimal bias for optimal demand prediction.
- Inventory Turnover Rate The inventory turnover rate measures how often inventory is sold and replaced over a given period. A higher turnover rate generally indicates that demand forecasting is more accurate, leading to better inventory management and reduced carrying costs. By tracking this metric, businesses can gauge how well they are managing stock levels based on forecasted demand.
Conclusion
Effective demand forecasting is vital for eCommerce businesses to optimize inventory, minimize costs, and boost customer satisfaction. By leveraging advanced tools and continuously tracking KPIs like forecast error and stockout rates, businesses can improve forecast accuracy and adapt to market fluctuations.
The right forecasting methods and real-time insights empower businesses to make data-driven decisions and remain competitive in the fast-paced eCommerce landscape.
Frequently Asked Questions
Q1. How can machine learning improve demand forecasting accuracy?
Machine learning algorithms analyze large volumes of historical data, recognize patterns, and predict future demand with higher precision. These models can also adapt to changes in demand dynamics, improving accuracy over time, especially for products with irregular sales patterns.
Q2. What role do external factors, like economic trends or weather, play in demand forecasting?
External factors significantly influence demand forecasting accuracy. By incorporating causal forecasting models, businesses can account for events such as economic shifts, weather changes, or social trends that affect consumer behavior and demand, thus improving the relevance of predictions.
Q3. How does demand sensing differ from traditional demand forecasting?
Demand sensing involves real-time data analysis and adjusts forecasts based on immediate market conditions, such as sales spikes or inventory shortages. Unlike traditional methods, which rely heavily on historical data, demand sensing helps businesses make short-term, dynamic adjustments to inventory and supply chain strategies.
Q4. What are the limitations of using historical data for demand forecasting?
While historical data is crucial, it may not fully account for significant shifts in market behavior, new product trends, or sudden external disruptions. Businesses should combine historical data with predictive analytics, machine learning, and real-time data to address these limitations and ensure more accurate forecasts.
Optimize Your Inventory Effortlessly
Receive timely insights and updates to ensure your inventory stays perfectly aligned with demand.
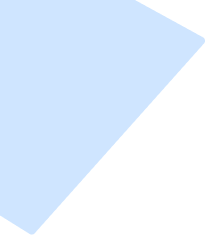
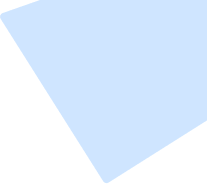

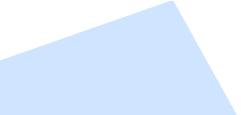